Uncertainty quantification in diffusion#
kinisi
is an open-source Python package that can accurately estimate diffusion processes in atomic and molecular systems and determine an accurate estimate of the uncertainty in these processes.
This is achieved by modelling the diffusion process as a multivariate normal distribution, based on that for a random walker.
This ensures and accurate estimation of the diffusion coefficient and it’s uncertainty.
More information about the approach kinisi
uses can be found in the methodology preprint article, which is also introduced in this poster.
kinisi
can handle simulation trajectories from many common molecular dynamics packages, including VASP and those that can be read by MDAnalysis.
Examples of some of these analyses are shown in the notebooks, also given there is an example of using kinisi
to investigate the Arrhenius relationship of diffusion as a function of temperature.
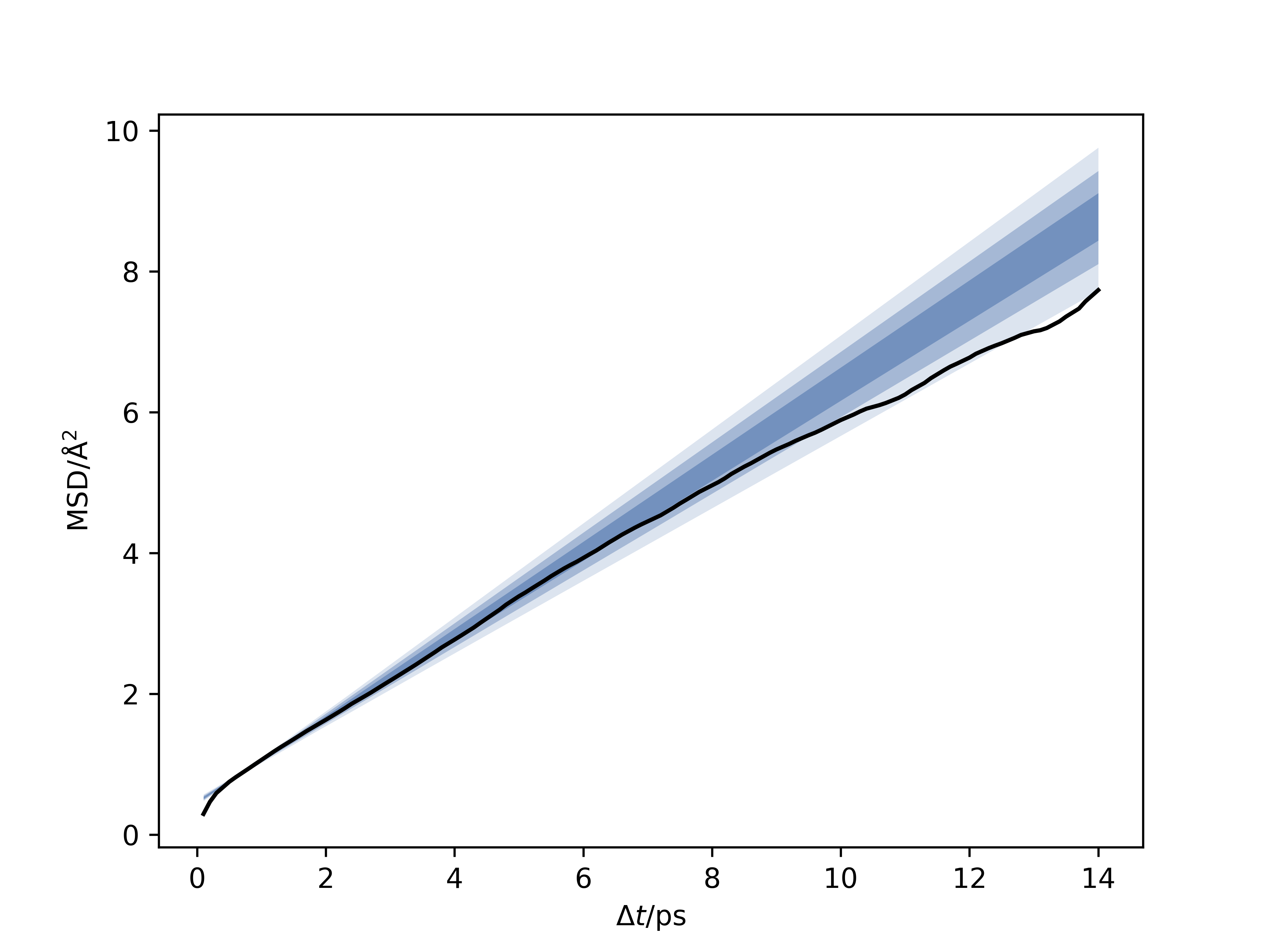

kinisi
; showing the determined mean-squared displacements (solid line),the estimated Einstein diffusion relationship (blue regions representing descreasing credible intervals).
Contributors#
Andrew R. McCluskey | Benjamin J. Morgan | Alex G. Squires | Josh Dunn